Tom Doyle
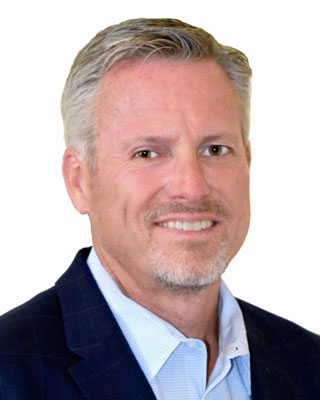
Want to Reduce Power in Always-on IoT Devices? Analyze First (2020)
Status: Available NowHundreds of millions of portable smart speakers are listening for a wake word. Millions more acoustic event-detection devices are listening for window breaks, baby cries or dog barks. Consumers appreciate how easy it is to use their always-on listening devices – but the battery drain that results from continuously processing all sounds in their environment? Not so much.
The problem is that this massive number of battery-powered IoT devices are notoriously power-inefficient in the way that they handle sound data. Relying on the age-old “digitize-first” system architecture, these devices digitize all the incoming sensor data as soon as they enter the device; then the data are processed for relevance, and in some cases, sent to the cloud for further analysis and verification. Since 80-90% of all sound data are irrelevant in most always-listening IoT devices, the digitize-first approach wastes significant battery life.
This session will show attendees how an “analyze first” edge architecture that uses analogML at the front end of an always-listening device eliminates the wasteful digitization and processing of irrelevant data, to deliver unprecedented power-saving and data efficiency in IoT devices.
Session attendees will:
- Understand that while most of today’s machine learning is implemented digitally, machine learning can also be implemented in ultra-low-power programmable analog blocks (analogML) so that feature extraction and classification can be performed on a sensor’s native analog data.
- Understand that the power problem for IoT devices is really a problem of the device treating all data as equally important and that determining which data are important earlier in the signal chain — while the data are still analog — reduces the amount of data that are processed through higher-power digital components. This approach saves up to 10x in system power in IoT devices.
- Learn how to integrate this new analogML edge architecture with sensors and MCUs from leading semiconductor suppliers into current and next-generation IoT devices.